Machine learning model uses blood tests to predict COVID-19 survival
Levels of 14 proteins in the blood of critically ill COVID-19 patients are associated with survival
A single blood sample from a critically ill Covid-19 patient can be analyzed by a machine learning model which uses blood plasma proteins to predict survival, weeks before the outcome, according to a new study published in the open-access journal PLOS Digital Health by Florian Kurth and Markus Ralser of the Charité – Universitätsmedizin Berlin, Germany, and colleagues.
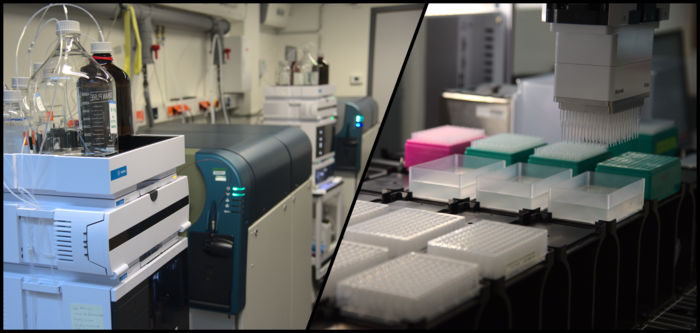
Proteomics core facility at Charité University hospital Berlin
Johannes Hartl, Charité
Healthcare systems around the world are struggling to accommodate high numbers of severely ill COVID-19 patients who need special medical attention, especially if they are identified as being at high risk. Clinically established risk assessments in intensive care medicine, such as the SOFA or APACHE II, show only limited reliability in predicting future disease outcomes for COVID-19.
In the new study, researchers studied the levels of 321 proteins in blood samples taken at 349 timepoints from 50 critically ill COVID-19 patients being treated in two independent health care centers in Germany and Austria. A machine learning approach was used to find associations between the measured proteins and patient survival.
15 of the patients in the cohort died; the average time from admission to death was 28 days. For patients who survived, the median time of hospitalization was 63 days. The researchers pinpointed 14 proteins which, over time, changed in opposite directions for patients who survive compared to patients who do not survive on intensive care. The team then developed a machine learning model to predict survival based on a single time-point measurement of relevant proteins and tested the model on an independent validation cohort of 24 critically ill COVID-10 patients. The model demonstrated high predictive power on this cohort, correctly predicting the outcome for 18 of 19 patients who survived and 5 out of 5 patients who died (AUROC = 1.0, P = 0.000047).
The researchers conclude that blood protein tests, if validated in larger cohorts, may be useful in both identifying patients with the highest mortality risk, as well as for testing whether a given treatment changes the projected trajectory of an individual patient.
Original publication
Other news from the department science
These products might interest you
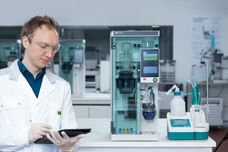
Kjel- / Dist Line by Büchi
Kjel- and Dist Line - steam distillation and Kjeldahl applications
Maximum accuracy and performance for your steam distillation and Kjeldahl applications
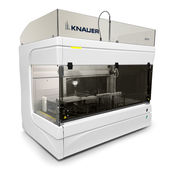
AZURA Purifier + LH 2.1 by KNAUER
Preparative Liquid Chromatography - New platform for more throughput
Save time and improve reproducibility during purification
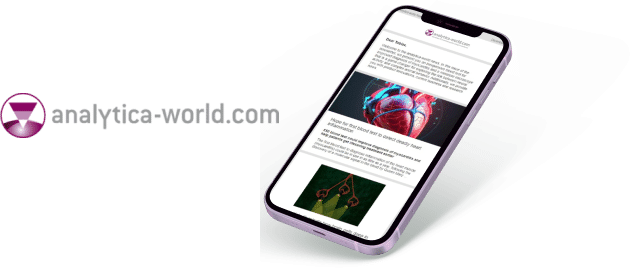
Get the analytics and lab tech industry in your inbox
From now on, don't miss a thing: Our newsletter for analytics and lab technology brings you up to date every Tuesday. The latest industry news, product highlights and innovations - compact and easy to understand in your inbox. Researched by us so you don't have to.